In this issue we have recommended reading on creating a modern data workspace, enterprise search & AI, enterprise AI trends, no-code / low-code companies, Medium’s latest pivot, Facebook’s new publishing platform plan, and Wikipedia getting the big guys to pay.
Our content technology news weekly will be out Wednesday as usual.
Decoding the no-code / low-code startup universe and its players
No-code and low-code companies are sprouting up everywhere and whatever your initial impression has been, it’s time to consider their increasing utility and role in even complex application development environments. No-code / low-code can democratize and speed development by bringing business analysts and software developers closer together. Pietro Invernizzi and Ben Tossell have put together a very helpful resource looking at 145 companies with lots of detail and access to an Airtable spreadsheet. (Click the image for a version you can read)
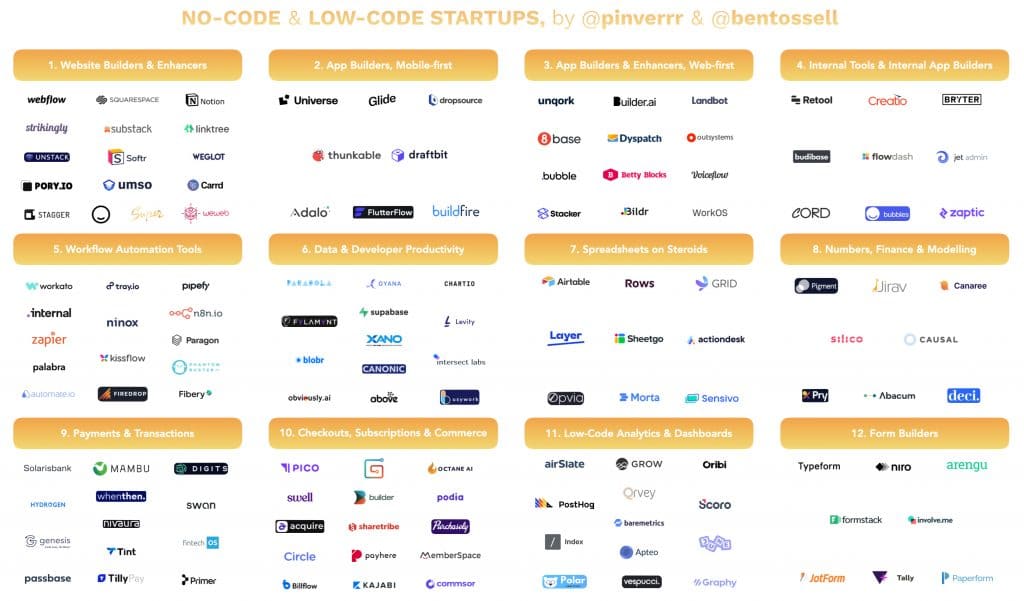
We failed to set up a data catalog 3x. Here’s why
Prukalpa Sankar generously and delightfully describes what she and her team learned from multiple attempts at creating a “modern data workspace”. Some of you will be familiar with the problems and lessons, but the approaches, tools used, and specific examples, will still be instructive.
When explainable AI meets enterprise search
Lack of transparency in AI is in general an unsolved problem even though there is obviously huge value in its application across domains. Martin White has some thoughts and advice on what this means for enterprise search.
Enterprise search presents a special challenge when it comes to AI transparency. Most other enterprise processes are close to linear in execution, so the impact of AI on performance can be relatively easily assessed and monitored. In the case of search, every query is a new workflow as it is dependent on the knowledge of the individual and the intent behind their search.
The mess at Medium
Lots of activity in the independent writing/publishing platform space recently: the Substack Pro controversy, Twitter’s acquisition of Revue, Facebook’s announcement of a new platform for independent writers, and Medium’s latest pivot. None of these companies has figured out a sustainable business model, and none provide writers a safe long-term way to control their brand and content. They can in some cases be supplemental marketing and delivery channels if you own and control your content and publishing capability however. 🙂
Casey Newton reports on the changes at Medium…
Medium’s original journalism was meant to give shape and prestige to an essentially random collection of writing, gated behind a soft paywall that costs readers $5 a month or $50 a year. Eleven owned publications covered food, design, business, politics, and other subjects… But in the end, frustrated that Medium staff journalists’ stories weren’t converting more free readers to paid ones, Williams moved to wind down the experiment…
Also worthy
- Facebook’s new platform… Supporting independent voices, via Facebook
- Great free teaser content as usual… Enterprise AI trends to watch In 2021 via CBInsights
- Wikipedia Enterprise… Wikipedia Is finally asking big tech to pay up via Wired
- Long but worthy article by Tim O’Reilly on the bigger picture… The end of Silicon Valley as we know it? via O’Reilly Radar
The Gilbane Advisor is curated by Frank Gilbane for content technology, computing, and digital experience professionals. The focus is on strategic technologies. We publish more or less twice a month except for August and December. We also publish curated content technology news weekly We do not sell or share personal data.
Subscribe | Feed | View online | Editorial policy | Privacy policy